[ad_1]
On Tuesday, the peer-reviewed journal Science published a study that exhibits how an AI meteorology mannequin from Google DeepMind referred to as GraphCast has considerably outperformed typical climate forecasting strategies in predicting world climate situations as much as 10 days upfront. The achievement means that future climate forecasting might turn out to be way more correct, reviews The Washington Post and Financial Times.
Within the examine, GraphCast demonstrated superior efficiency over the world’s main typical system, operated by the European Centre for Medium-range Climate Forecasts (ECMWF). In a complete analysis, GraphCast outperformed ECMWF’s system in 90 % of 1,380 metrics, together with temperature, strain, wind velocity and path, and humidity at numerous atmospheric ranges.
And GraphCast does all this rapidly: “It predicts lots of of climate variables, over 10 days at 0.25° decision globally, in beneath one minute,” write the authors within the paper “Studying skillful medium-range world climate forecasting.”
This marks a notable development in velocity and accuracy for AI in meteorology. Matthew Chantry, machine-learning coordinator at ECMWF, acknowledged the speedy progress in an interview with the Monetary Instances, saying that an AI system in meteorology had progressed “far sooner and extra impressively than we anticipated even two years in the past.”
GraphCast makes use of what researchers name a “graph neural community” machine-learning structure, educated on over 4 a long time of ECMWF’s historic climate information. It processes the present and six-hour-old world atmospheric states, producing a 10-day forecast in a few minute on a Google TPU v4 cloud laptop. Google’s machine studying technique contrasts with typical numerical climate prediction strategies that depend on supercomputers to course of equations based mostly on atmospheric physics, consuming considerably extra time and vitality.
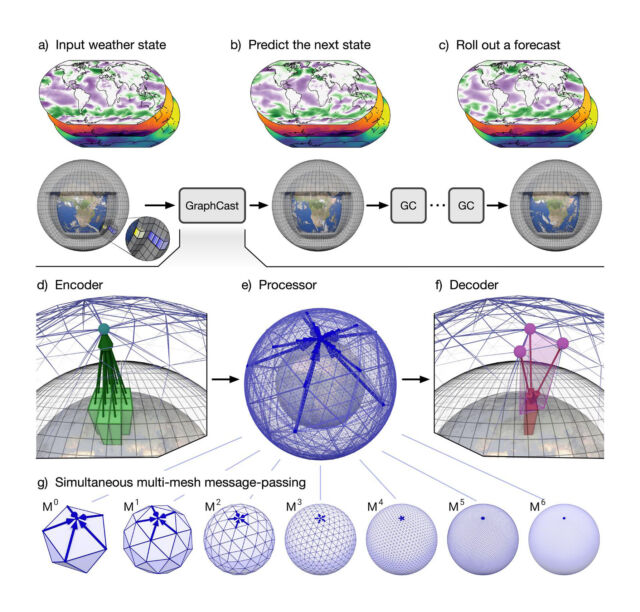
Google DeepMind
Chantry highlighted GraphCast’s effectivity to the Monetary Instances, estimating it to be about 1,000 occasions cheaper when it comes to vitality consumption in comparison with conventional strategies. An instance of its forecasting success was its prediction of Hurricane Lee’s landfall in Nova Scotia 9 days upfront, three days sooner than conventional approaches.
Regardless of the development, GraphCast has limitations. It didn’t outperform typical fashions in all situations, such because the sudden intensification of Hurricane Otis, which hit Acapulco with minimal warning on October 25. Additionally, as a consequence of technological limitations, world AI fashions cannot but create forecasts as detailed or granular as conventional ones, making them extra perfect for inspecting smaller-scale phenomena, in response to The Washington Publish. They usually have transparency points since meteorologists cannot but look contained in the “black field” of the AI mannequin and see precisely why it makes the forecast it does.
Finally, the Google DeepMind researchers see their AI-based method as a complement to present climate prediction strategies. “Our method shouldn’t be thought to be a alternative for conventional climate forecasting strategies,” they write, “which have been developed for many years, rigorously examined in lots of real-world contexts, and provide many options we’ve not but explored.”
Trying forward, ECMWF plans to develop its personal AI mannequin and discover integrating it with its numerical climate prediction system. The UK Met Workplace, in partnership with the Alan Turing Institute, can be growing a graph neural community for climate forecasting to be integrated into its supercomputer infrastructure sooner or later.
[ad_2]
Source link